
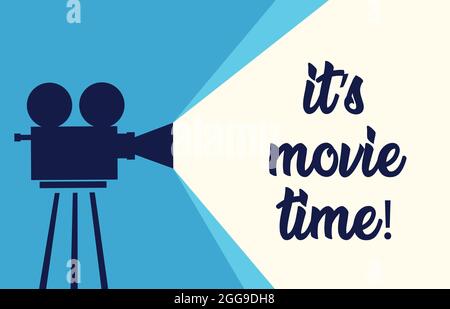
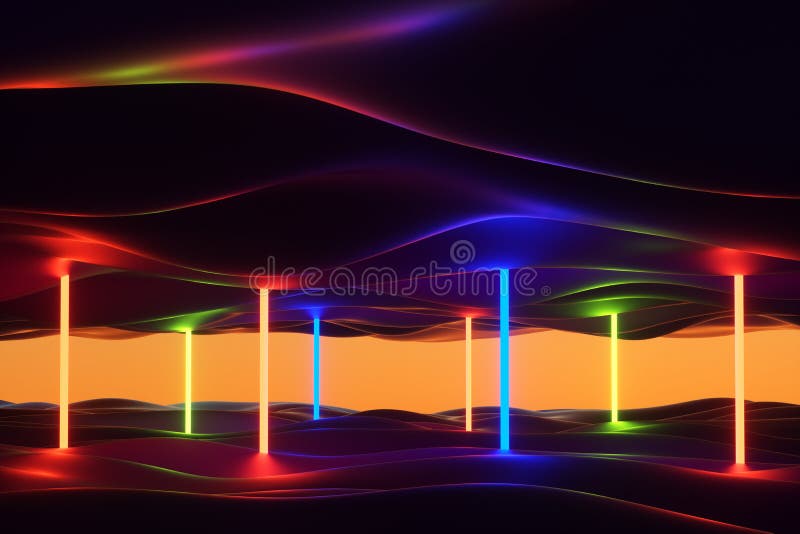
This semantic approach takes the problem space as the starting point for the description: the domain for which data is acquired. This approach goes beyond the traditional description of the structure of the data warehouse: a semantic approach is required. The processing of data is therefore not only a logistical challenge, but also requires a reliable approach to map the meaning of data. The introduction of a distributed data architecture provides the final push to “clean up the attic of data”. The lack of available data specialists makes it necessary to make available knowledge explicit. Additionally, the increasing variety of data calls for a grip on the individual data sources. Data lineage – the traceability of data to its meaning and the reason for which the data is used – is becoming a critical success factor. The interest in the meaning of data is increasing. Which data governance model can lead to the best results.Which technological solutions are needed to set up good data management.Why it is important to look not only at internal but also at external data.How more accurate data can contribute to better algorithms.

#BEAM MODELSTORMER TEMPLATE HOW TO#
How to increase the “data literacy” of your employees.Which aspects are really relevant to get value from your data.What are the key elements that need to be put in place to ensure success? How do you move from a technology-centric to an integrated data strategy? How do we improve the data literacy of the stakeholders and ensure that the data products can be used effectively? With the regularity of the clock, we introduce new concepts such as data fabric and data mesh, where the question remains to what extent they solve problems or introduce new problems. Too many organizations still fail to actually get value from their data initiatives. The real question has now shifted to ensuring that the value is delivered in a sustainable way. It’s no longer about convincing management of the value of using data to create business value.
